Active Research Domains/Projects
Pathological Hand Tremor Extraction for Robotic Rehabilitation Systems
Pathological hand tremor (PHT) is among the most common movement symptoms of several neurological disorders including Parkinson’s Disease (PD) and Essential Tremor (ET). Extracting PHT is of paramount importance in several engineering and clinical applications such as assistive and robotic rehabilitation technologies. In such systems, PHT is modeled as the input noise to the system and thus there is a surge of interest in estimation an compensation of the noise.
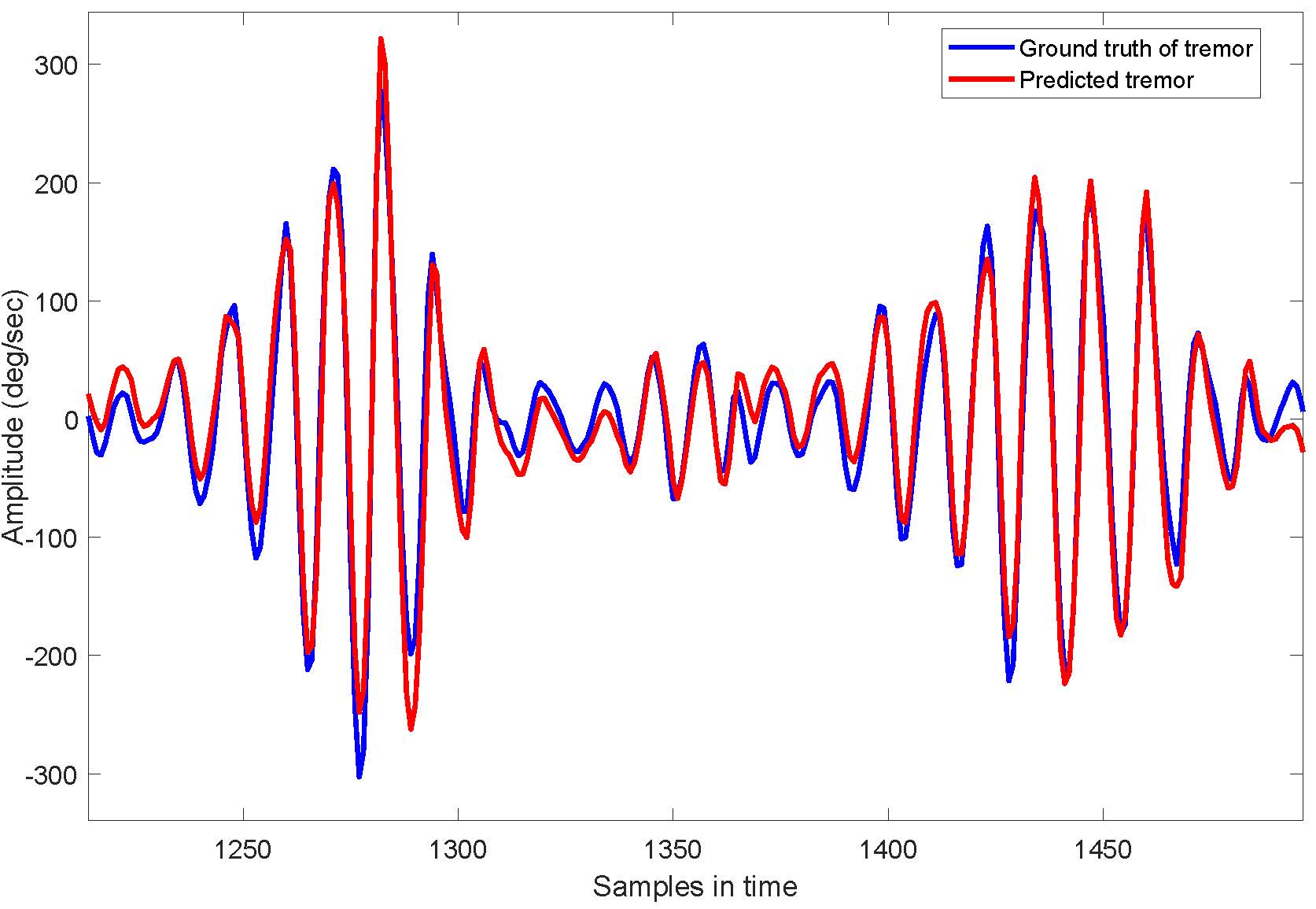
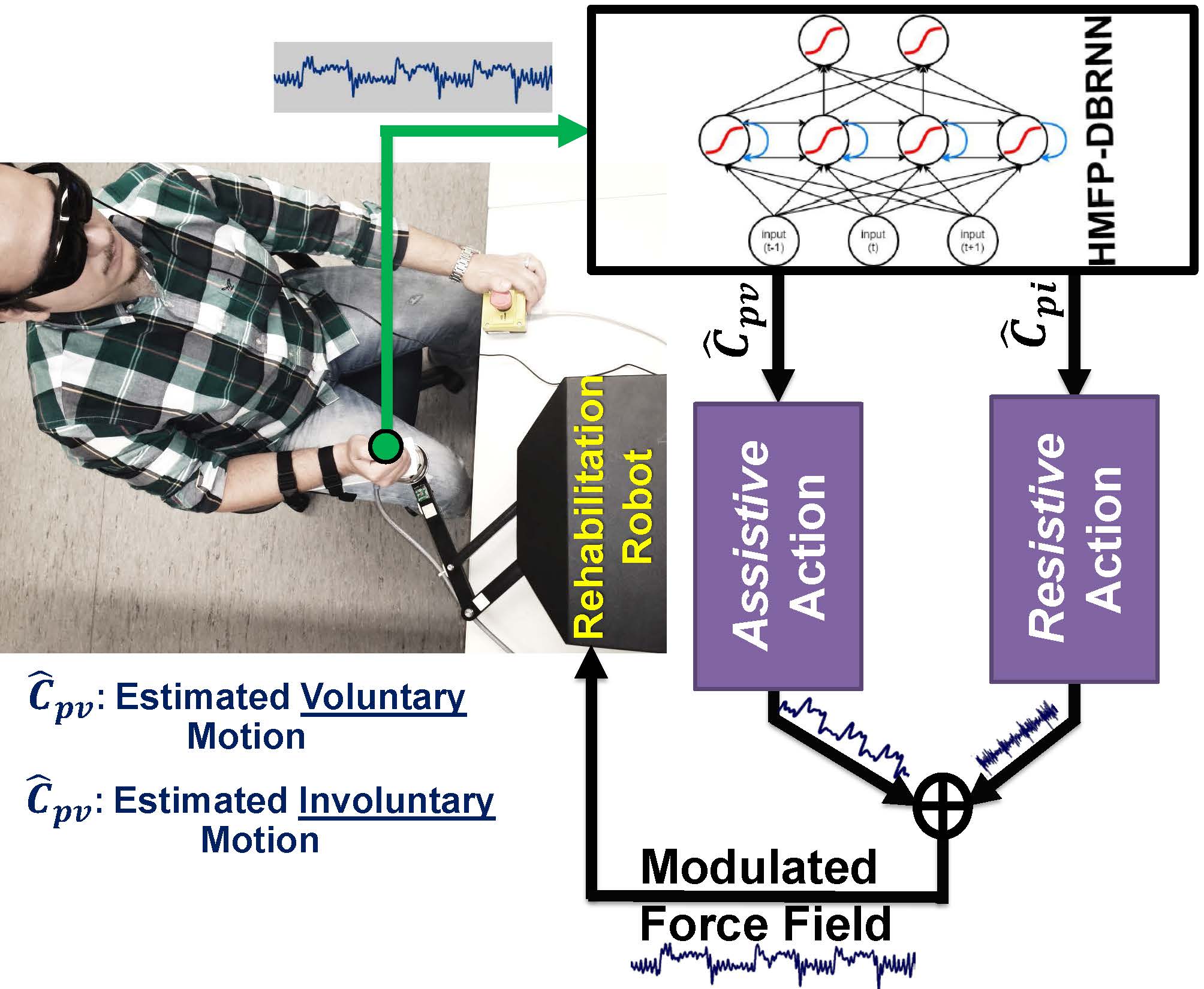
The tremor is considered as a critical measure for physicians to perform diagnosis and to recommend appropriate treatment therapies. Having a tremor extraction algorithm with high accuracy is, therefore, both beneficial and essential to differentiate common movement disorders such as Parkinson’s disease and Essential Tremor, because in many cases they can result in hand tremor with similar characteristics.
In rehabilitation and assistive settings such as robotic rehabilitation systems and active assistive technologies, accurate and real-time tremor extraction with minimum phase lag is crucially necessary to allow for proper delivery of rehabilitative and assistive actions and guarantee the required level of safety.
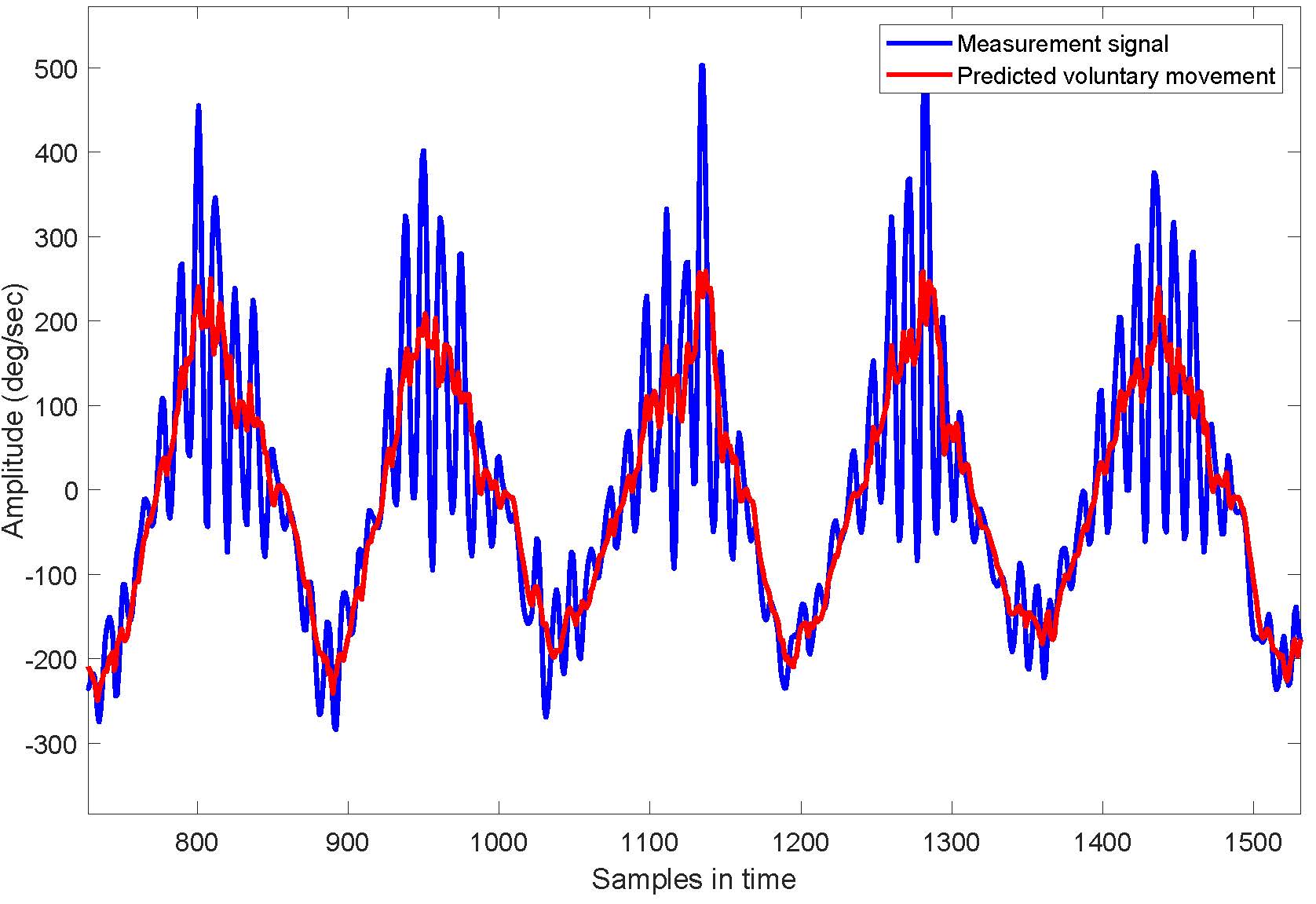
2017 SMC Conference
2018 BIO Symposium
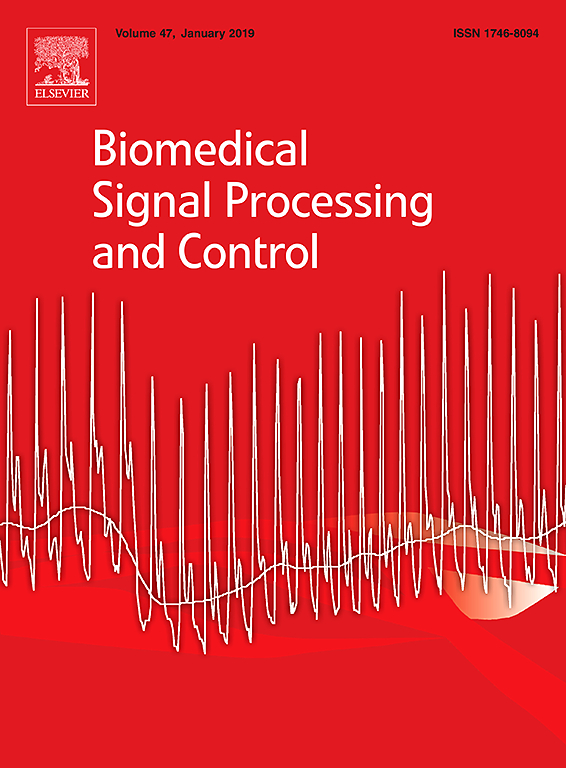
WAKE: Wavelet Decomposition Coupled with Adaptive Kalman Filtering for Pathological Tremor Extraction,
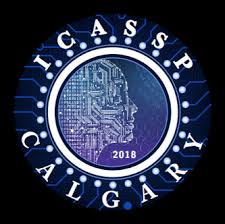
Multiple-Model and Reduced-Order Kalman Filtering for Pathological Hand Tremor Extraction,
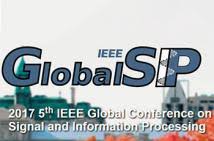
Dynamic Estimation Strategy for E-BMFLC Filters in Analyzing Pathological Hand Tremors,
Brain Computer Interfacing (BCI)
Despite recent advances in signal processing, machine learning, and computational technologies, our brain remains unparalleled in its information processing abilities to analyze and fuse different multimodal, streaming signals in an adaptive and real-time fashion. A unique characteristic of human brain is its plasticity property, i.e., the ability of neurons to modify their behaviour (structure and functionality) in response to environmental diversity. This exclusive property has recently been utilized by researchers to recover the ability of moving legs in patients paralyzed with spinal cord injuries after training them with an exoskeleton linked to their brain. The plasticity property of brain has also motivated design of brain-computer interfaces (BCI) to develop an alternative form of communication between human brain signals and the external interfacing world. BCIs have several therapeutic applications of significant importance to help partially or fully-disabled patients interact with outside world, including but not limited to rehabilitation/assistive systems, rehabilitation robotics, and neuro-prosthesis control.
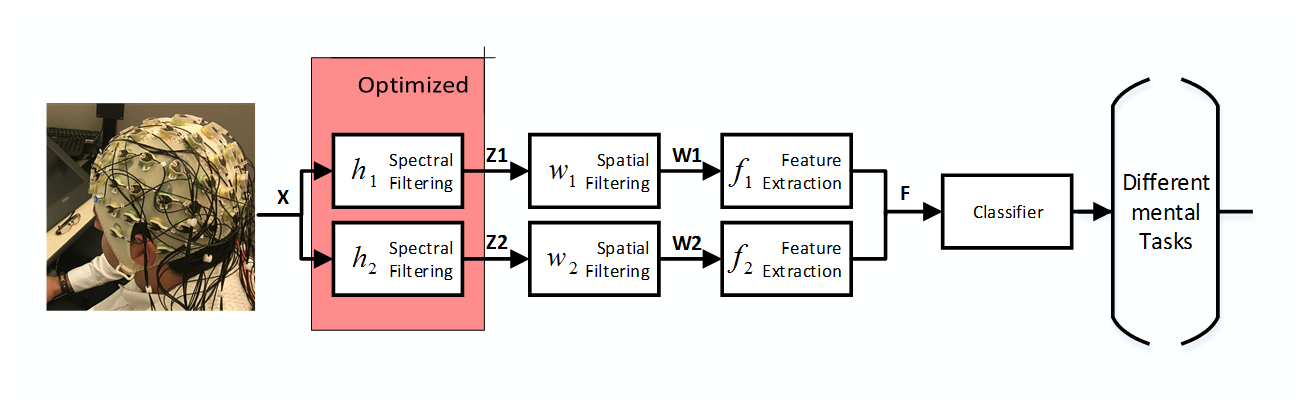
In this work, we consider problem of classifying multiple Motor Imagery (MI) tasks by means of Error Correcting Output Coding (ECOC) coupled with Common Spatial Patterns (CSP) technique. The Double Bound Bayesian spatio-spectral filter optimization (B2B) deploys a Bayesian framework to optimize two spectral filters applied to the EEG signals.
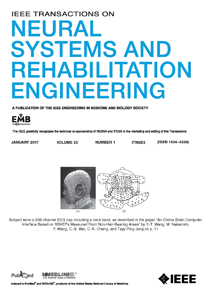
Optimized Double Band Spectra-Spatial Filters Coupled with Ternary ECOC for BCIs,
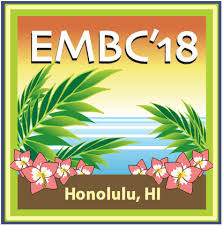
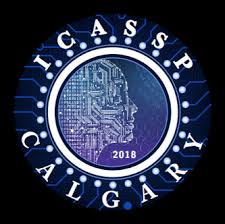
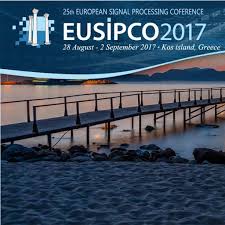
Error correction output codding coupled with the CSP for motor imagery BCI systems,
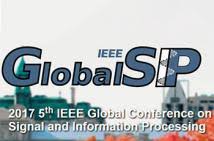
Adaptive Dimensionality Reduction Method using Graph-based Spectral Decomposition for Motor Imagery-based Brain-Computer Interfaces.,
Event-based Estimation/Control
In response to recent advancements of cyber-physical systems, event-based estimation (EBE) strategies have emerged as an innovative alternative to the conventional time-driven state estimation schemes. In particular, EBE approaches address issues related to limited bandwidth; satisfy power constraints of a remote sensor, which cannot afford to periodically transfer its measurements to the fusion centre (FC) via utilization of an equidistant sampling sachem, and reduce communication overhead (the main source of sensor’s power consumption) by capitalizing on the fact that the information content of a remote sensor changes from time to time. In the EBE approaches, measurements from a sensor to the estimator are communicated only in occurrence of specific events identified based on a local triggering mechanism implemented at the sensor level. The basic motivation behind development of EBE algorithms is to reduce the communication overhead by avoiding periodic transfer of measurements.
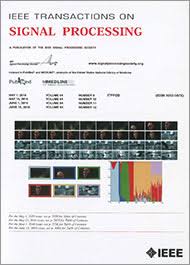
Event-Based Estimation With Information-Based Triggering and Adaptive Update,
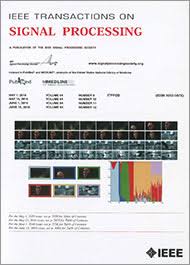
CEASE: A Collaborative Event-triggered Average-consensus Sampled-data framework with Performance Guarantees for Multi-agent Systems,
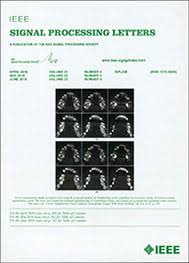
Ternary-Event-Based State Estimation With Joint Point, Quantized, and Set-Valued Measurements,
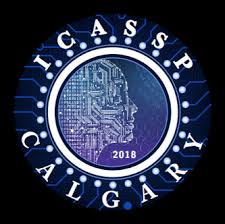
Event-Triggered Particle Filtering Via Diffusion Strategies for Distributed Estimation in Autonomous Systems,
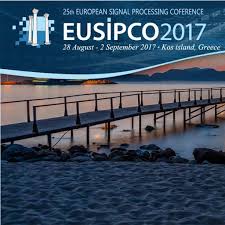
Event-based Particle Filtering with Point and Set-valued Measurements,
WAKE-BPAT: Blood Presure Estimation
The paper is motivated by recent urgency to design continuous and cuff-less blood pressure (BP) monitoring solutions to prevent, detect, and treat the hypertension. In this regard, we propose a novel wavelet-based feature extraction algorithm coupled with an adaptive and multiple-model Kalman filtering framework (referred to as the WAKE-BPAT), which provides accurate and dynamic BP estimates by extraction and fusion of different pulse arrival time (PAT) features. In particular, a wavelet transform and histogram analysis based robust and high-accurate R-peak detection algorithm is proposed without incorporation of any pre-defined thresholds.
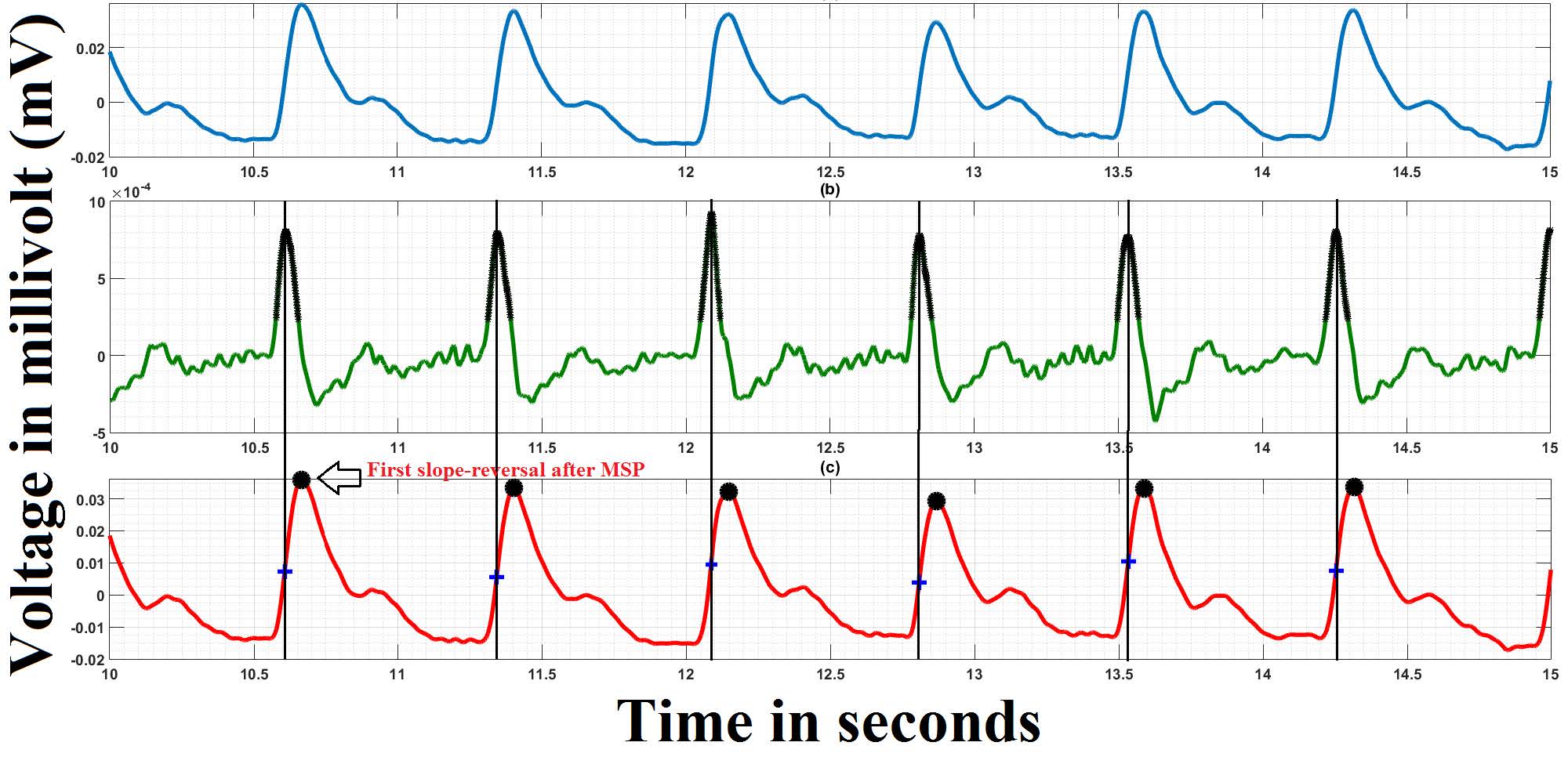
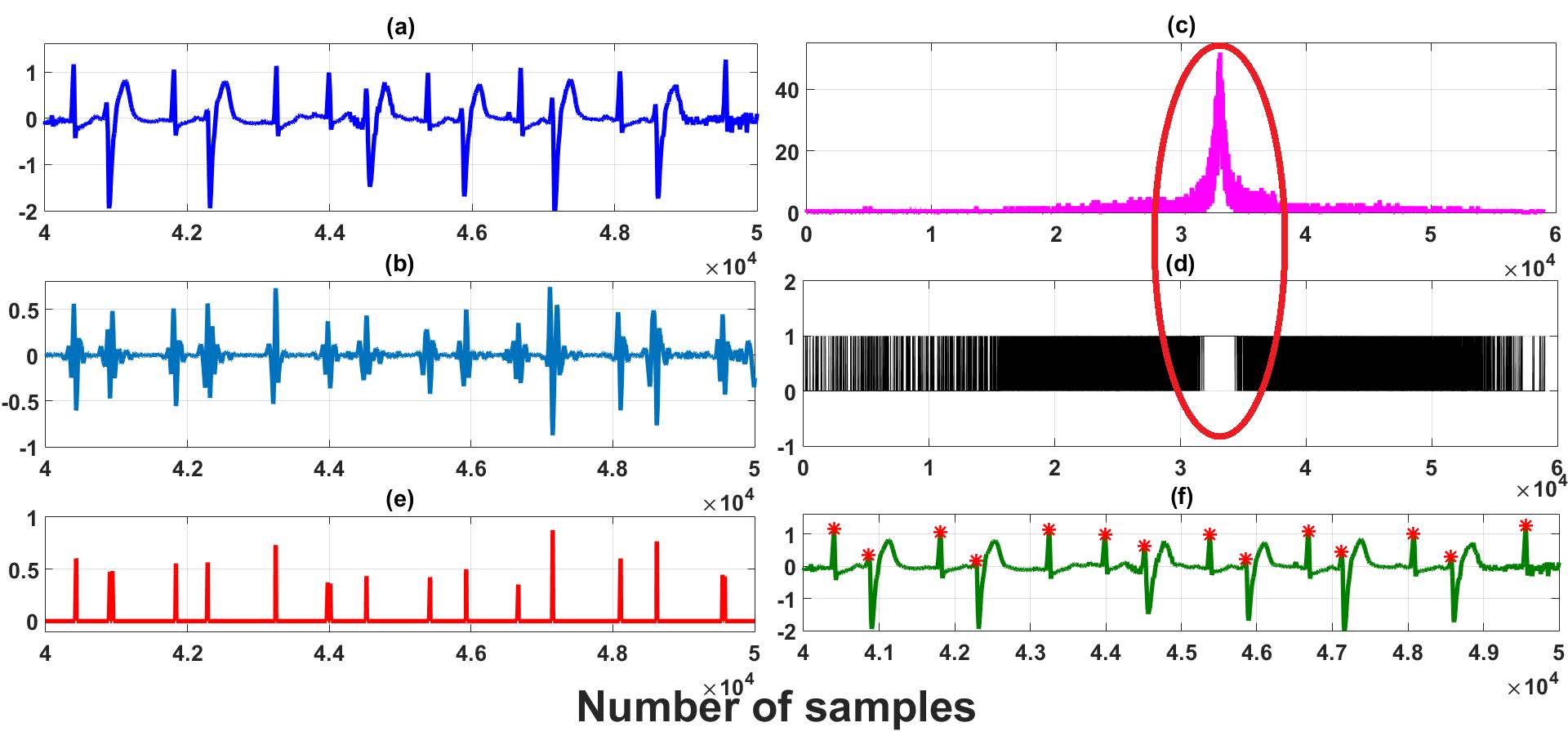
This in combination with high-quality photoplethysmogram (PPG) characteristic points obtained from signal recordings of a recently developed PPG device (Gen-1), are used for BP estimation, which is modeled as a hybrid state-space model with structural uncertainties to fuse different PAT features in an adaptive fashion. Our experimental evaluations based on a real data set collected via Gen-1 device confirms the superiority of the proposed WAKE-BPAT framework in comparison to its counterparts.
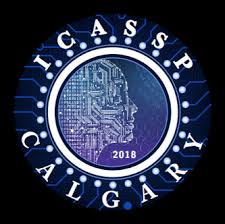
WAKE-BPAT: Wavelet-Based Adaptive Kalman Filtering for Blood Pressure Estimation Via Fusion of Pulse Arrival Times,
Lung Cancer Radiomics
Recent advancements in signal processing coupled with developments of electronic medical record keeping in hospitals and the availability of extensive set of medical images through internal/external communication systems, have resulted in a recent surge of significant interest in "Radiomics". Radiomics is an emerging and relatively new research field, which refers to extracting semi-quantitative and/or quantitative features from medical images with the goal of developing predictive and/or prognostic models, and is expected to become a critical component for integration of image-derived information for personalized treatment in near future. The conventional Radiomics workflow is typically based on extracting pre-designed features (also referred to as hand-crafted or engineered features) from a segmented region of interest. Nevertheless, recent advancements in deep learning have caused trends towards deep learning-based Radiomics. Capitalizing on advantageous and disadvantageous of these two approaches, there are also hybrid solutions developed to exploit the potentials of multiple data sources. Considering these variety of approaches to Radiomics, further improvements require a comprehensive and integrated sketch, which is the goal of this research.